Unlocking Insights: How https://www.informaticsview.com Empowers Your Data-Driven Journey
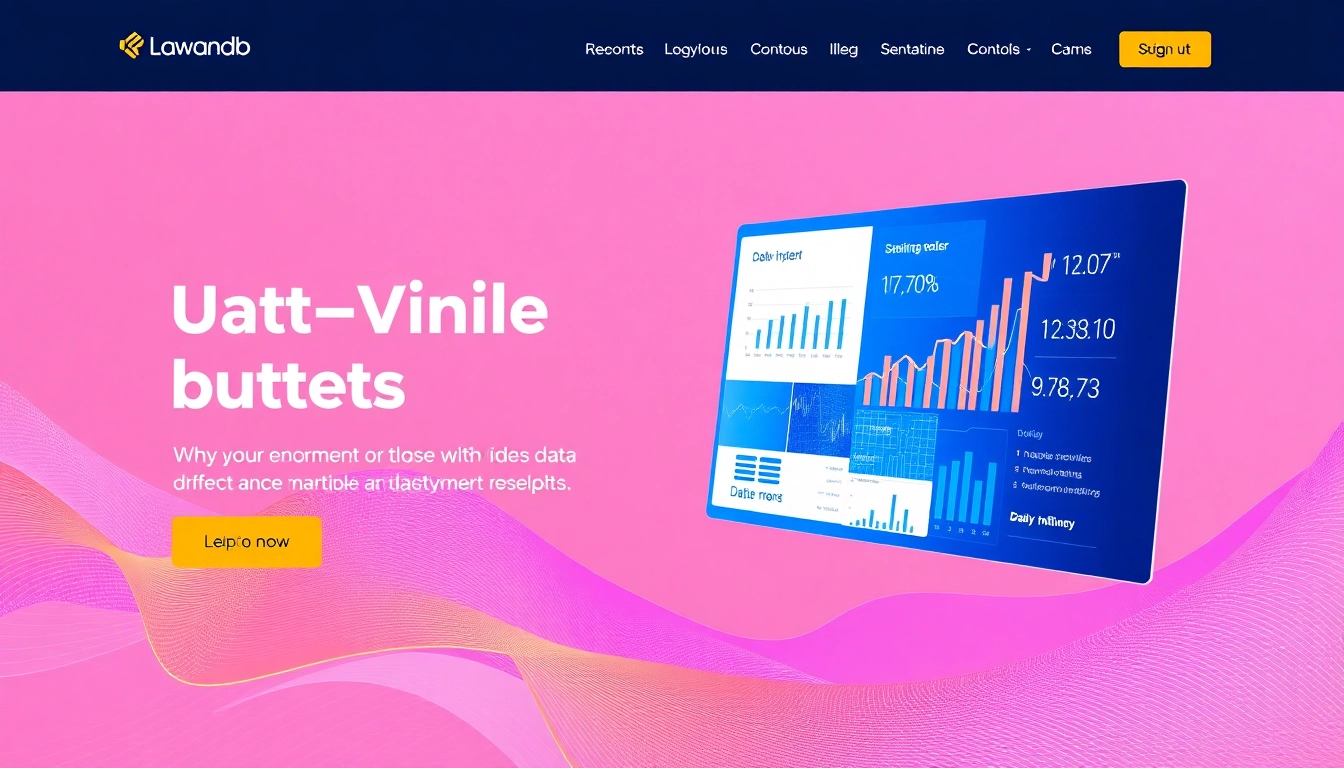
Understanding Data-Driven Insights
What are Data-Driven Insights?
Data-driven insights refer to information derived from the systematic analysis of data. In today’s digital landscape, organizations collect vast amounts of data from various sources—customer interactions, market trends, operational processes, and more. Analyzing this data allows businesses to uncover patterns, correlations, and trends that inform strategic decisions. Data-driven insights are essential for optimizing performance, enhancing user experience, and minimizing costs. Essentially, they transform raw data into actionable recommendations that can guide everything from marketing strategies to product development.
Benefits of Leveraging Data
The primary advantage of leveraging data-driven insights is enhanced decision-making. By grounding decisions in empirical evidence rather than intuition, organizations can reduce risks and increase the likelihood of successful outcomes. Some of the key benefits include:
- Improved Accuracy: Data-driven decision-making enhances the precision of strategies by relying on quantifiable evidence.
- Increased Efficiency: Analyzing data helps identify inefficiencies within processes, leading to quicker and smarter workflows.
- Better Customer Insights: Organizations can better understand their customers’ needs and preferences, allowing for more personalized experiences.
- Competitive Advantage: Companies that utilize data effectively can predict market shifts and respond proactively, giving them an edge over competitors.
Key Concepts in Data Analysis
To effectively harness data-driven insights, it is vital to grasp key concepts in data analysis:
- Descriptive Analytics: This form of analysis helps summarize past data to identify trends and patterns.
- Predictive Analytics: These techniques utilize statistical algorithms and machine learning to forecast future outcomes based on historical data.
- Prescriptive Analytics: This advanced analysis offers recommendations for actions by evaluating potential outcomes.
- Data Visualization: This refers to representing data graphically to simplify complex information and improve comprehension.
Strategies for Effective Data Utilization
Adopting a Data-First Mindset
For organizations to maximize the benefits of data analytics, they must cultivate a data-first mindset across all levels. This involves creating a culture that values data and promotes its use in decision-making processes. Key steps to adopt this mindset include:
- Leadership Support: Management should advocate for data-driven approaches and invest in necessary tools and technologies.
- Training Programs: Providing staff with training on data literacy ensures that more team members can interpret and utilize data effectively.
- Cross-Department Collaboration: Data insights can provide value across departments, so fostering collaboration ensures comprehensive data utilization.
Tools and Resources for Data Analysis
Several tools and resources are available that can streamline the data analysis process. Choosing the right combination will depend on specific business needs. Some popular options include:
- Google Analytics: Essential for understanding website performance, user interaction, and traffic sources.
- Tableau: A powerful data visualization tool that helps users create interactive dashboards.
- R and Python: Programming languages heavily used in data analysis and statistical computing.
- Excel: While often underestimated, Excel remains a versatile tool for data analysis and visualization.
Case Studies from https://www.informaticsview.com
To demonstrate the impact of data-driven practices, several case studies illuminate successful strategies adopted by various organizations. For instance:
- Retail Sector: A large retail chain implemented predictive analytics to forecast inventory needs, which resulted in a 15% reduction in stock-outs.
- Healthcare: A healthcare provider employed data visualization tools to track patient outcomes, leading to improved treatment plans and patient satisfaction rates.
- Finance: A bank used customer data to identify cross-selling opportunities, resulting in a significant increase in overall revenue.
Common Challenges in Data Analytics
Identifying Data Quality Issues
Data quality issues can significantly impair the utility of insights derived from analysis. Organizations often struggle with data that is incomplete, outdated, or inaccurate. Common symptoms include:
- Inconsistent data formats.
- Missing records or fields.
- Duplicate entries in datasets.
Addressing these issues involves implementing strict data governance practices to ensure data integrity and accuracy through regular audits and cleansing practices.
Navigating Complex Data Regulations
As data becomes more integral to business practices, navigating regulatory frameworks such as GDPR and HIPAA can prove challenging. These regulations ensure the protection of consumer information but can complicate data collection and analysis efforts. Organizations must:
- Stay informed about relevant regulations.
- Invest in compliance tools and software.
- Train employees on best practices for data handling.
Data Silos and Their Impact
Data silos occur when departments or business units within an organization do not share their data with one another. This can lead to inefficiencies and a lack of comprehensive insights. Overcoming silos requires:
- Establishing centralized data repositories.
- Encouraging interdepartmental communication and collaboration.
- Implementing integrated data management systems to streamline access to data across the organization.
Best Practices for Data Management
Establishing Data Governance
Data governance involves defining policies and procedures to manage data availability, usability, integrity, and security. Good governance helps organizations utilize data as a strategic asset. Best practices for establishing data governance include:
- Defining data ownership and accountability.
- Creating a data management framework that aligns with organizational goals.
- Regularly reviewing and updating policies to reflect changes in data practices.
Implementing Data Security Measures
Protecting data from breaches and unauthorized access is paramount. Organizations should adopt best practices for data security, including:
- Using encryption and secure access protocols.
- Conducting regular security audits and assessments.
- Implementing role-based access controls to limit data access to authorized personnel only.
Ensuring Data Accessibility for Teams
While security is crucial, data should still be accessible to those who need it to make informed decisions. Achieving a balance requires:
- Implementing user-friendly data management interfaces.
- Providing training for employees on how to access and utilize data.
- Encouraging collaboration through shared dashboards and visualization tools.
Measuring Success in Data-Driven Initiatives
Setting Performance Metrics
To gauge the effectiveness of data-driven initiatives, organizations need to establish clear performance metrics. These metrics should directly align with business objectives and could include:
- Sales growth attributable to insights generated from data analysis.
- Time saved in process optimizations driven by data.
- Customer satisfaction scores linked to personalized service initiatives.
Analyzing Results and Insights
Once performance metrics are established, analyzing results is crucial to understand the impact of data-driven strategies. This involves:
- Regularly reviewing data and reports to identify trends.
- Adjusting strategies based on findings to enhance effectiveness.
- Engaging stakeholders in interpreting the results to foster a shared understanding of successes and areas for improvement.
Continuous Improvement Strategies
Success in data-driven initiatives is not a one-time effort but requires ongoing commitment. Continuous improvement can be achieved by:
- Regularly revisiting and revising data strategies based on emerging technologies and trends.
- Encouraging feedback from teams who utilize data in their processes.
- Investing in training to ensure that staff can keep pace with advancements in data analytics.
Leave a Comment